Overview
The Bantay Bangga project investigates correlations between road crash injuries of varying severity (e.g., minor, serious, and fatal) and hospital capacity (for some definition of "capacity" involving bed occupancy, equipment availability, and staff manpower).
The analysis relies on two sources of data:
approximately 46,000 road incident records in the Philippines spanning from 2016 to 2024
approximately one million records of hospital bed occupancy, human resources, and medical equipment from the Department of Health (DOH) from 2020 to 2022 during the height of the COVID-19 pandemic.
Bantay Bangga is a data science project undertaken in fulfillment of CS 132 under Professor Paul Regonia, during the second semester of the academic year 2023-2024 at the University of the Philippines Diliman.
Background
The past decade has seen an alarming 39% increase in road-related deaths from 2011-2021, according to the 2023 Global Status Report on Road Safety by the Department of Transportation (DOTr) alongside the World Health Organization (WHO).
Increase in annual road casualties in the PH
7938
in 2011
11096
in 2021
Road injuries cost approx.
2.6%
of the country's GDP
In response, DOTr and WHO had devised the Philippine Road Safety Action Plan. This plan seeks to reduce annual road traffic deaths by 35% by the year 2028 by focusing on five aspects:
Road safety management | enhancing research, gaining the trust of stockholders, adopting global best practices |
---|---|
Safer roads | improving infrastructure, road maintenance, addressing the needs of vulnerable road users (e.g., cyclists, motorcyclists, pedestrians, children, elderly, PWD) |
Safer vehicles | enhancing vehicle registration, inspection, and regulation in compliance with vehicle standards |
Safer road users | increasing public awareness of road safety, enforcement of government laws |
Post-crash response | improving access and timeliness to care and rehabilitation |
Action Plan
We examine the injury types of recorded road incidents starting from the onset of the COVID-19 pandemic in 2020 to its slumps in 2022.
We then relate the number of minor, serious, and fatal injuries with different factors of hospital capacity (e.g., bed occupancy, staff availability, and equipment availability) using statistical analysis with hypothesis testing.
We train an ordinary least-squares (OLS) regression model to predict the number and severity of road incident injuries given the state of the nationwide hospital capacity at that time.
Research Questions
To what extent does hospital capacity affect the fatality rate of road incidents?
What factors contribute to a hospital's overall capacity to provide care to patients?
Data Collection
Road Crash Incidents
Source
Obtained from the Project CREATE SQL databases of the Intelligent Transport Systems Laboratory (ITSLab) at the National Center for Transportation Studies (NCTS).
As of writing, the data set includes incidents from 2016 up to 2024, amounting to 46,209 total road incidents.
Hospital Capacity
during the COVID-19 Pandemic
Source
Obtained from the DOH Data Drops, maintained and regularly updated by the DOH on patient and hospital information during the COVID-19 pandemic.
The data sets collected for this study span from July 2020 to November 2022, amounting to 1.4 million rows of daily reports from medical facilities across the country.
Data Exploration
Weekly Trends
Road Injury Types by Week
The road incident data set is first aggregated by each recorded date, accounting for the number of minor injuries, serious injuries, fatal injuries, and total injuries on that day. The rate of each incident severity is also computed using the number of incidents of a particular severity over the total number of incidents. The data is then aggregated by week and by month.
Bed Occupancy Percentage by Week
The hospital bed occupancy data is aggregated as a percentage: occupied beds over the total beds (sum of occupied and vacant). The data is then aggregated by week and by month by getting the mean occupancy percentage for the week/month.
Average Medical Staff Availability by Week
The medical staff data is aggregated as a sum per day, and by the mean per week and per month.
Total Medical Equipment by Week
The medical equipment data is aggregated as a sum per day, week and month.
Research Question 1: To what extent does hospital capacity affect the fatality rate of road incidents?
Bed Occupancy vs. Minor Injuries
First, the total number of road crash victims with minor injuries is compared to the total bed occupancy. Eyeballing the plot shows a slight linear relationship between the two variables with a correlation coefficient and a -value to be . However, these results are unfortunately not statistically significant at a significance level of 90%. That is, we fail to reject the null hypothesis: the total number of minor injuries is not linearly related to the total bed occupancy.
However, a multivariate linear regression shows more promising results. The best and simplest set of predictors involve the beds occupied by COVID-19 patients. Specifically, these two variables are:
- The total number of COVID-19 patients in (ICU) beds
- The total number of COVID-19 patients in non-ICU beds
These two variables yield with the -values and , respectively. At a significance level of 95%, the null hypothesis may therefore be rejected with statistical certainty. That is, the total number of COVID-19 patients in ICU beds and non-ICU beds form a linear combination that determines the total number of minor injuries in road crash incidents.
Bed Occupancy vs. Serious Injuries
A similar analysis can be done for serious injuries. Plotting the weekly total number of beds versus the weekly total number of serious injuries yields the scatter plot above. The regression results in with a -value of . Again, these results are not statistically significant. We thus fail to reject the null hypothesis: these two variables are not linearly related.
Unfortunately, searching for a stronger correlation involving COVID-19 patients and ICU beds (just like in the previous section) leads to the same negative results. There is no statistically significant way to linearly relate the weekly total bed occupancy with the total weekly number of serious injuries in road crash incidents.
Bed Occupancy vs. Fatal Injuries
For fatal injuries, the correlation with hospital capacity is stronger. With the weekly total number of occupied beds being the metric for hospital capacity, we obtain a (negative) correlation with and a -value of . At a significance level of 95%, we may reject the null hypothesis with statistical certainty: the two variables are indeed linearly related. Contrary to our initial hypotheses, when the weekly total number of occupied beds increase, the weekly total number of fatal road crash incidents decreases.
Meanwhile, with the weekly total number of occupied ICU beds by COVID-19 patients as the metric for hospital capacity, we obtain a (negative) correlation with and a -value of . At a significance level of 95%, we may reject the null hypothesis with statistical certainty: the two variables are indeed linearly related. In other words, when the weekly total number of occupied ICU beds (by COVID-19 patient) increase, the weekly total number of fatal injuries in road crash incidents decrease.
Finally, with the weekly total number of occupied non-ICU beds by COVID-19 patients as the metric for hospital capacity, we obtain a negative correlation with with a -value of . At a significance level of 95%, we may reject the null hypothesis with statistical certainty: the two variables are indeed linearly related. In other words, when the weekly total number of occupied non-ICU beds (by COVID-19 patient) increase, the weekly total number of fatal injuries in road crash incidents decrease.
Bed Occupancy vs. Total Injuries
Staff Resources vs. Minor Injuries
Plotting the weekly total number of staff members (i.e., doctors, nurses, and support staff) versus the weekly total number of minor injuries from road crash incidents yields with a -value of . From these results alone, it is apparent that there is no linear relationship between these two variables. A similar analysis into the weekly total number of doctors, nurses, and support staff (individually) yields no better conclusions. That is, staff resources are not a good predictor for the weekly total number of minor injuries in road crash incidents.
Staff Resources vs. Serious Injuries
Plotting the weekly total number of staff members (i.e., doctors, nurses, and support staff) versus the weekly total number of serious injuries from road crash incidents yields with a -value of . From these results alone, it is apparent that there is no linear relationship between these two variables. A similar analysis into the weekly total number of doctors, nurses, and support staff (individually) yields no better conclusions. That is, staff resources are not a good predictor for the weekly total number of serious injuries in road crash incidents.
Staff Resources vs. Fatal Injuries
Plotting the weekly total number of staff members (i.e., doctors, nurses, and support staff) versus the weekly total number of fatal injuries from road crash incidents yields with a -value of . From these results alone, it is apparent that there is no linear relationship between these two variables. However, a similar analysis into the weekly total number of doctors, nurses, and support staff (individually) yields better conclusions in multivariate linear regression.
With the weekly total number of doctors as the metric for hospital capacity, we obtain a positive correlation with and a -value of . At a significance level of 95%, we may reject the null hypothesis with statistical certainty: the two variables are indeed linearly related. Interestingly, when the weekly total number of doctors increase, so do the weekly total number of fatal injuries in road crash incidents. An alternate interpretation is that the weekly total number of nurses increase when the weekly total number of fatal injuries also increase (to meet the demand).
With the weekly total number of nurses as the metric for hospital capacity, we obtain a positive correlation with and a -value of . At a significance level of 95%, we may reject the null hypothesis with statistical certainty: the two variables are indeed linearly related. Interestingly, when the weekly total number of nurses increase, so do the weekly total number of fatal injuries in road crash incidents. An alternate interpretation is that the weekly total number of nurses increase when the weekly total number of fatal injuries also increase (to meet the demand).
Medical Equipment vs. Minor Injuries
Here, the total number of road crash victims who sustained minor injuries is compared to the weekly total medical equipment. Looking at the plot would reveal a slight linear relationship between the two variables with a correlation coefficient of and a -value of . These results are unfortunately insufficient to conclude any statistical significance given our proposed significance level of 95%. Thus, for this, we fail to reject the null hypothesis: the total number of minor injuries is not linearly related to the weekly total medical equipment.
Looking at the multivariate linear regression did not conclude any promising results, with there being no predictors (i.e., specific medical equipment) that was within the desired significance level. There is no statistically significant way to linearly relate the weekly total medical equipment with the total weekly number of minor injuries in road crash incidents.
Medical Equipment vs. Serious Injuries
Plotting the total number of road crash victims with serious injuries in relation to weekly total medical equipment shows a linear relationship between the two, with a correlation coefficient of and a -value of . These results fall under the proposed 95% significance level set by the researchers, and thus we reject our null hypothesis. In fact, opposite to what was predicted by the hypotheses, as the total number of medical equipment increases, the number of weekly total road crash incidents increase as well.
Following that, a multivariate linear regression confirms our predictions with some interesting and promising results. More specifically, the predictors being gloves, face shields, and surgical masks all scored well within the 95% significance level with -values of , , and , respectively. They scored values of , , and , respectively.
These three variables are also well-within the 95% significance level, meaning the weekly total of gloves, face shields, and surgical masks all form a linear combination that determines the total weekly number of serious injuries in road crash incidents.
Medical Equipment vs. Fatal Injuries
Plotting the weekly total number of medical equipment (i.e., gown
, gloves
, head_cover
, goggles
, coverall
, shoe_cover
, face_shield
, surgmask
, and n95mask
) versus the weekly total number of fatal injuries from road crash incidents yields with a -value of . From the results alone, it is apparent that there is no linear relationship between these two variables. A similar analysis into weekly totals of each individual equipment type yields no better conclusions. That is, medical equipment are not good predictors for the weekly total number of fatal injuries in road crash incidents.
Research Question 2: What factors contribute to a hospital's overall capacity to provide care to patients?
As seen in the previous section, there are several factors that contribute to a hospital's overall capacity to provide care to its patients.
During the pandemic, the bed occupancy of COVID-19 patients is one of the more statistically significant predictors for the eventual severity of road crash injuries (namely that of minor and fatal injuries).
Meanwhile, the total number of medical staff plays little to no role when predicting the severity of injuries. The only exception occurs with fatal injuries, where the weekly total number of doctors and nurses are statistically significant predictors.
Finally, the available medical equipment also plays little to no role when predicting the severity of injuries. The only exception occurs with serious injuries, where the weekly total number of gloves, surgical masks, and face shields interestingly are statistically significant predictors.
Modelling with Ordinary Least-Squares Linear Regression
From the data, certain linear trends are apparent. To supplement the findings above, an ordinary least-squares regression model (OLS) is trained to predict the number of injuries when given a subset of hospital capacity features (e.g., bed occupancy, medical staff readiness, and medical equipment availability). As an application of the project's findings, this model is in line with the research questions raised earlier.
Predicting the Minor Injuries
The first OLS model attempts to predict the total weekly number of minor injuries given the nationwide hospital capacity for that week. The baseline (null) model is one that always predicts the mean of the entire weekly aggregation, which is . Hence, the null model has a root mean-squared error (RMSE) of (i.e., the "score" to beat as a baseline).
In accordance with the findings in the previous section, the selected features for hospital capacity are:
- the weekly total number of ICU beds occupied by COVID-19 patients (
icu_o_c
); - the weekly total number of vacant beds dedicated to COVID-19 patients (
total_covid_v
); - and the weekly total number of used mechanical ventilation units (
mechvents_used
).
It turns out that this subset of features yields the least RMSE out of the other subsets that have been exhaustively tried. A 10-fold cross-validation of the model reports as the RMSE and as the score. Note that the RMSE of the OLS model is better than that of the null model. Unfortunately, the negative score is an indicator for a poorly fitted model, but this is the best that can be done with the data.
Predicting the Serious Injuries
The second OLS model attempts to predict the total weekly number of serious injuries given the nationwide hospital capacity for that week. The baseline (null) model uses the mean . Hence, the null model has a root mean-squared error (RMSE) of (i.e., the "score" to beat as a baseline).
In accordance with the findings in the previous section, the selected features for hospital capacity are:
- the weekly total number of ICU beds occupied by non-COVID-19 patients (
icu_o_nc
); - the weekly total number of available beds (both ICU and non-ICU) (
total_beds_v
); - the weekly total number of vacant mechanical ventilation units (
total_mechvent_v
); - and the weekly total number of available surgical masks (
surgmask
).
A 10-fold cross-validation of the model reports an RMSE of and an score of given this subset of features. An exhaustive search over all possible feature subsets shows that this yields the best metrics. Just like in the previous model, although the OLS model performs better than the null model, the slightly negative score indicates a poorly fitted model.
Predicting the Fatal Injuries
The final OLS model attempts to predict the total weekly number of fatal injuries given the nationwide hospital capacity for that week. The baseline (null) model uses the mean . Hence, the null model has a root mean-squared error (RMSE) of (i.e., the "score" to beat as a baseline).
In accordance with the findings in the previous section, the selected features for hospital capacity are:
- the weekly total number of occupied beds (both ICU and non-ICU) (
total_beds_o
); - the weekly total number of used mechanical ventilation units (
mechvents_used
); - the weekly total number of available surgical masks (
surgmask
); - and the weekly total number of available N95 masks (
n95
).
A 10-fold cross-validation of the model reports an RMSE of and an score of given this subset of features. An exhaustive search over all possible feature subsets shows that this yields the best metrics. Just like in the previous model, although the OLS model performs better than the null model, the slightly negative score indicates a poorly fitted model.
Simulations
Minor Injury Predictions
Given a total of:
- occupied ICU beds with COVID-19 patients
- vacant beds dedicated for COVID-19 patients
- or mechanical ventilation unit usage rate
The number of predicted minor injuries given the model was .
Serious Injury Predictions
Given a total of:
- occupied ICU beds with non-COVID-19 patients
- total vacant beds (inclusive of both ICU and non-ICU beds)
- vacant mechanical ventilation units
- available surgical masks
The number of predicted serious injuries given the model was .
Fatal Injury Predictions
Given a total of:
- occupied beds (inclusive of ICU and non-ICU beds)
- or mechanical ventilation unit usage rate
- available surgical masks
- available N95 masks
The number of predicted serious injuries given the model was .
Conclusions
Features That Matter
The researchers have noticed that upon using machine learning and linear regression, as well as looking into correlations between each feature, that the occupancy of hospital beds can affect the injury rates of road-related incident victims across all injury types (minor, serious, fatal). The vacancy of hospital beds, which was intriguing as that would mean the amount of hospital beds, indifferent to whether or not they're occupied, can be attributed to different road injury types.
Similarly, some medical equipment also proved to be worth looking into. These were namely the two main types of masks used during the COVID-19 pandemic, which are surgical and N95 masks, and medical ventilation machines/units. These equipment may prove vital in reducing road-related incident fatalities in the near future. This might hint at the possibility of needing more equipment for the near future, especially when hospitals are tending to a certain sickness at the time.
Interestingly, no features under the medical staff dataset were deemed to be related to road-related incidents. For every injury type, bed occupancy and equipment availability proved to be more influencing than hospital staff availability. This may mean that the count of hospital staff is sufficient for aiding road victims.
Looking Forward
The absence of the medical staff in the predictors may signify a need to focus more on supplying hospital resources rather than hiring more people. This indicates that medical staff quantity, and availability in general, is not the main factor behind road-related injuries and fatalities. Hiring more people isn't the solution.
ICU bed occupancy is a major predictor worth considering and looking into, as it is present in all three injury types' list of predictors. This may hint at the importance of hospital decongestion, which may result in patients with certain illnesses and injuries gaining more priority over road victims. It may also be worth noting for hospitals and governments in distributing and allocating patients, should there be multiple hospitals in the vicinity.
Several features and data prediction techniques were not touched upon by the researchers. Further studies and work may be directed towards the effect of hospital proximity and distance from each other, as well as the distance from the road event to the hospital. More advanced models such as neural networks may also be used for a more in-depth and possibly more accurate prediction. Some features and terms including "hospital capacity" could also be separated into multiple features and therefore defined better.
Notably, the researchers conclude that, based from the model, "hospital capacity" (for tending after road crash victims) does not significantly rely on the availability of hospital staff. Perhaps more data is required to determine if this were true or not, as it seems counter-intuitive that more room for patients would not need more staff tending to their needs.
Pilipinas in a Nutshell (PILIPINUTS 2023)
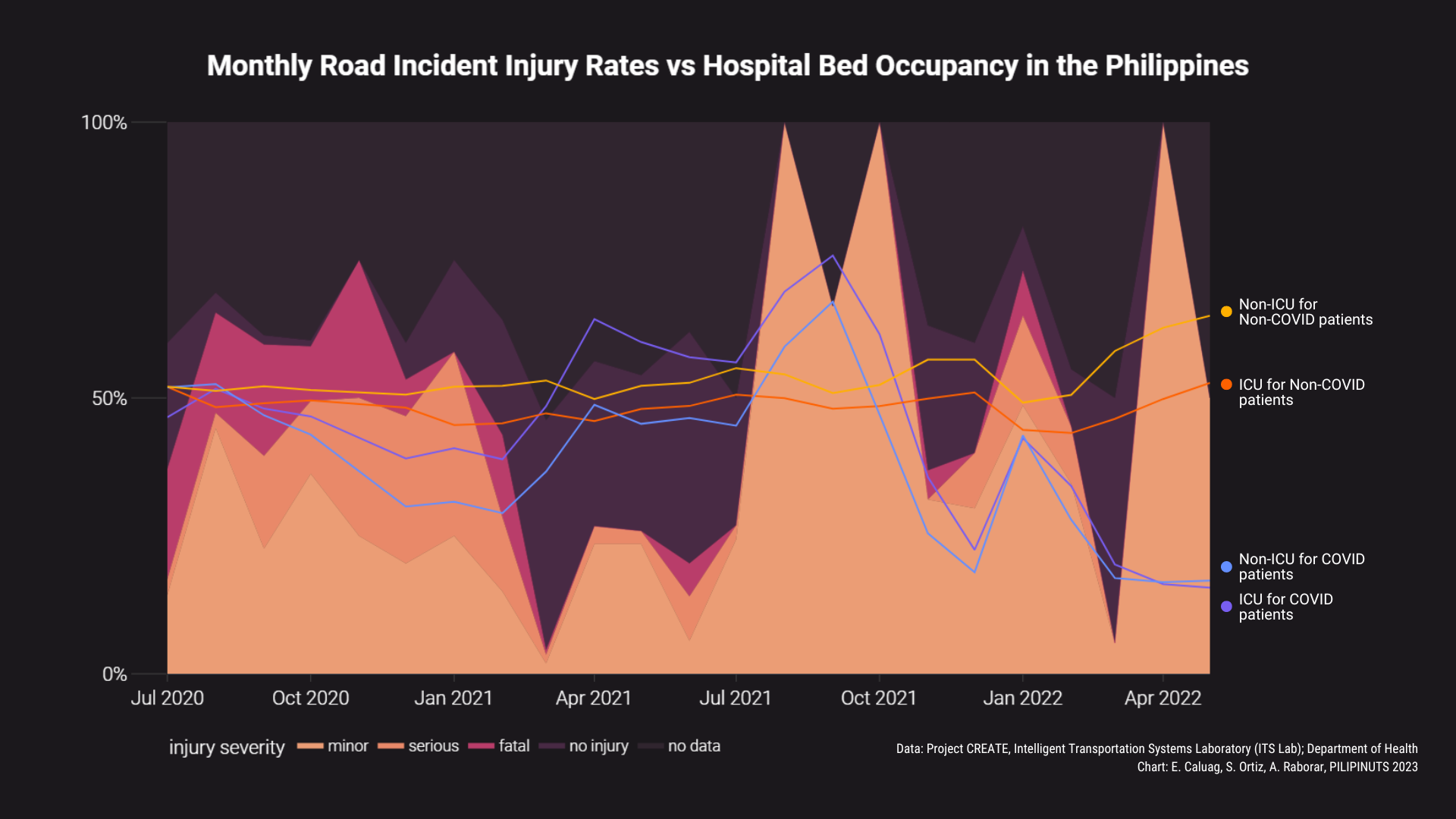
Road incidents in the Philippines are commonly reported. A fatality rate of 25% of incidents was recorded in November 2020, in addition to widespread cases of minor and serious injuries resulting from road incidents. To avoid unnecessary fatalities, hospital capacity and quality of care should be kept at a sufficient level for these road crash victims.
However, as the COVID-19 pandemic demanded the world's full attention, this unique situation poses an interesting inquiry into what could possibly affect road crash incident fatalities and their possible avenues for recovery in a time where something else (i.e., the pandemic) was the top priority.
Throughout the data analysis, the following features were of interest to the researchers: hospital bed occupancy (with COVID-19 and non-COVID-19 beds being noted), hospital staff availability, and medical equipment availability. A significance level of 95% was set as the threshold for deciding on whether to consider linear relationships between features of hospital capacity and road-related fatality rates.
Among these features, minor and fatal injuries are related to the hospital bed occupancy through a linear combination consisting of the total number of COVID-19 patients in both ICU beds and non-ICU beds, which possibly hints at the larger role of beds in hospital decongestion. Unfortunately, staff resources showed no linear relationship with any of the injury types and cannot be considered a predictor. Perhaps more data is required here. Meanwhile, medical equipment is linearly related with serious injuries through a linear combination of surgical masks, gloves, and face shields.
These aforementioned features are used in an OLS regression model to predict the weekly total number of minor, serious, and fatal injuries. A 10-fold cross-validation of the trained model confirms that it performs better than the null model (which just predicts the mean at all times). However, the coefficient of determination is mostly negative, which hints at a possibly poorly fit model. Further work can expound on the feature set by considering hospital proximity to the road crash incident or use an outright different machine learning model altogether (i.e., neural networks).
About Us
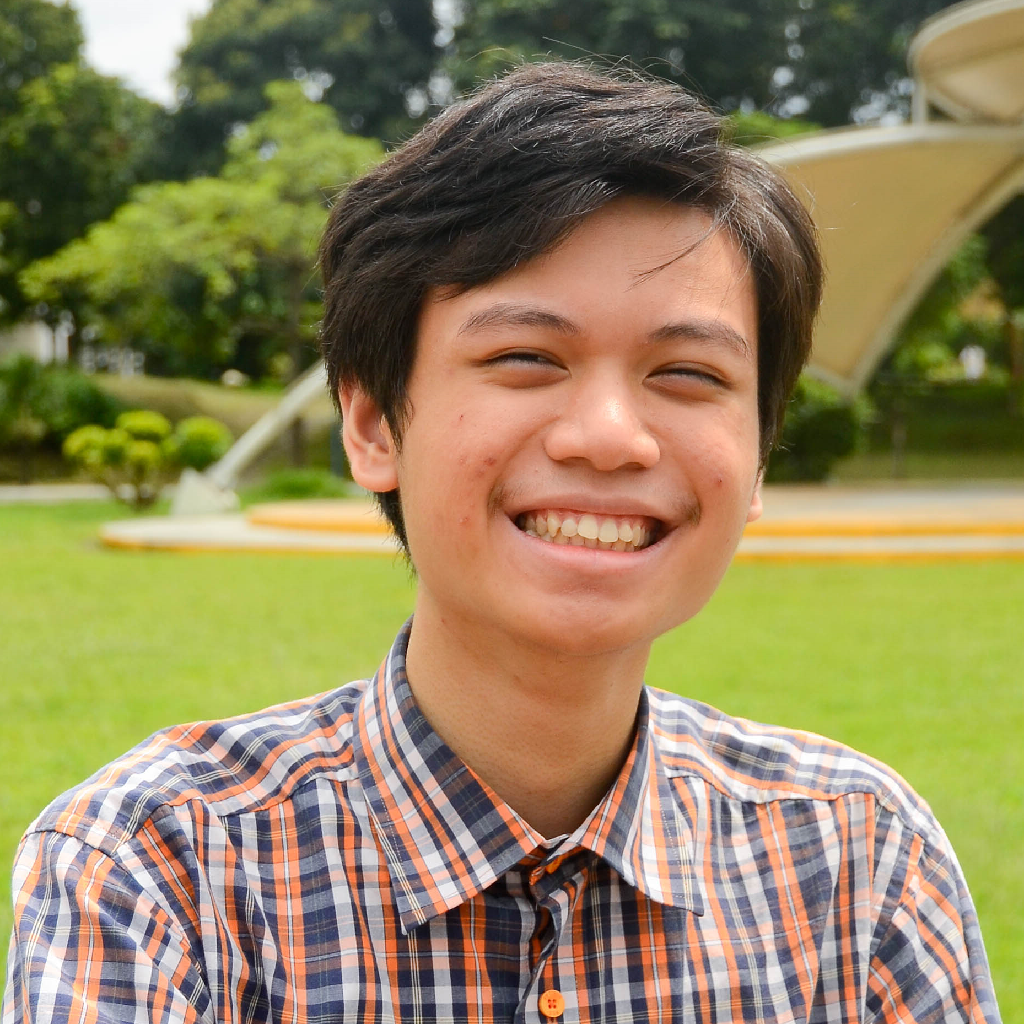